Application of Machine Learning Methods in Analyzing Customer Preferences in Grocery Store Chains
Keywords:
Customer preferences, machine learning, Classification, ClusteringAbstract
The primary objective of this research is to discover valuable patterns in customer data from grocery store chains to identify customer preferences and detect valuable customer churn. Using data mining and machine learning methods, valuable customers were identified based on RFM variables. This was achieved through clustering methods. Subsequently, valuable customers were classified using classification methods. The preferences of high-value customers were then identified through an association rule approach. Additionally, clustering and classification methods were employed to find useful patterns for analyzing the behavior of churned and non-churned customers. Following a series of data preparation and preprocessing operations, the data for each customer, along with their transactions, was determined. The initial data was based on each customer's transactions. However, after data preparation and preprocessing, datasets were created, capturing information specific to each customer. Using data mining and machine learning methods, alongside the three approaches of association rules, classification, and clustering, models and useful behavioral patterns were trained and constructed from the customer dataset of grocery store chains. Ultimately, a set of patterns and rules was developed to identify customer preferences, recognize valuable customers, and detect churned customers.
Downloads
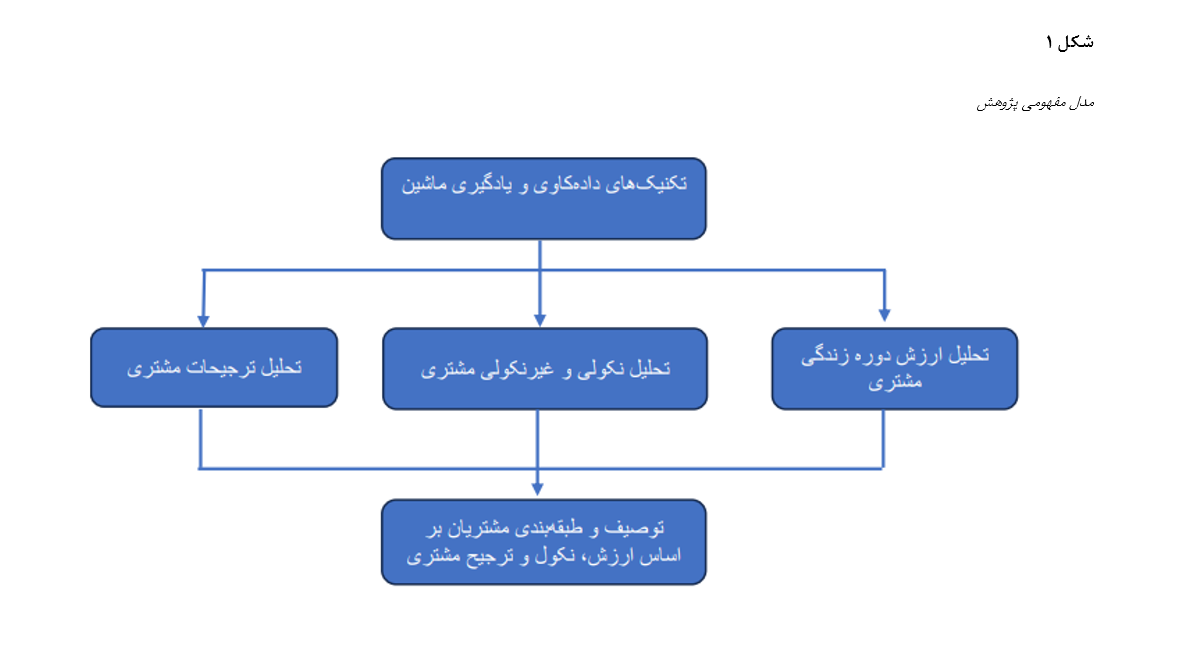